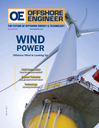
Page 12: of Offshore Engineer Magazine (May/Jun 2020)
Read this page in Pdf, Flash or Html5 edition of May/Jun 2020 Offshore Engineer Magazine
DRILLING & COMPLETIONS
AUTOMATED
MAINTENANCE REVIEW
TO BOOST PROJECT
EFFICIENCY AND SAFETY
By Chris Bell and Matthew Celnik, DNV GL - Oil & Gas apid increases in the development and deploy-
SWIFT, SMART AND THOROUGH ment of machine learning (ML) technologies in By quickly analyzing hundreds of maintenance records in just
E&P is offering offshore operators the chance a few seconds, the algorithms can deliver an effective means of to automate high-cost, error-prone tasks which determining relevant audit fndings and irregularities. This in- can adversely impact safety or production. creases project effciency for the team and allows more accurate
R
For instance, as part of the asset and verifcation mainte- fndings and recommendations to be made as part of the audit.
nance review process, it is standard practice to review text As opposed to a random sample method currently used by records for insights - with a focus trending defects and identi- most companies, ML can detect anomalies in the way main- fying improvements. The goal is to ensure the asset is perform- tenance has been recorded i.e. fail records marked as pass, ing safely and effectively, with high reliability, while adopting allowing the reviewer to focus purely on those which have the most cost-effective strategies for all maintenance work. inconsistencies. Equally, such techniques can be applied on
To better analyze a large number of records in full, one so- datasets for which analysis is repetitive, labor-intensive, or lution is to use an ML classifcation algorithm with natural prone to human error.
language processing. Asset owners can then analyze the trends across the entire set of maintenance records for each safety and
POSITIVES AND PITFALLS OF ML environmental critical element (SECE) on the asset (Figure 1). As an example, DNV GL considered a single fre and gas
Figure 1: Web tool developed by DNV GL to review free-text maintenance records using ML Figure 2: Confusion matrix showing ML accuracy against manually verifed training and testing data 12 OFFSHORE ENGINEER OEDIGITAL.COM