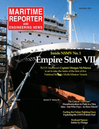
Page 15: of Maritime Reporter Magazine (September 2023)
Marine Design Edition
Read this page in Pdf, Flash or Html5 edition of September 2023 Maritime Reporter Magazine
learning researchers to show that if the data were available, the delity predictions in other seaways. This is what makes hybrid model could perform well, but data is almost never available methods incredibly powerful: they leverage data beyond its to the quantity and quality required. Furthermore, the need for traditional limits.
a large training data set means that despite the fast evaluation Some might argue that the highest-? delity, most sophisti- time of a data-driven method, the time to develop training data cated solution is not always the most pro? table. With good could be on a similar order of magnitude as a physics-based engineering judgment, the low-? delity, traditional approach analysis. And of course, if we spend the time to generate data can keep engineering costs low and still yield a relatively using a physics-based method, what is the value of the resulting high-quality product. This notion is certainly one of the big- data-driven model? To make data-driven methods viable, the gest hurdles when evaluating and adopting new methods, and training cost and the evaluation cost must be less than or equal hybrid methods are no exception. While it may be true that the to the evaluation cost of a physics-based method. bene? t a hybrid method can bring to an analysis is limited to
If physics-based methods are good, and data-driven methods certain applications, there is also the ever-increasing demand are fast, it is natural to wonder if they could be used in tandem for design performance: whether it be speed, seaway oper- to make the “fast and good” models that will greatly bene? t en- ability, survivability, safety, ef? ciency, serviceability, ease gineering analysis. But the solution takes more ingenuity than and economy of manufacturing, or environmental impact. It just training a neural network with a physics-based method. The may not be unreasonable to expect that the most performant reality is that training data requirements and a lack of transfer- design requirements will bene? t from the high-? delity data- ability will make such an approach of little help to an engineer leveraging bene? ts of a hybrid method. working on a novel design. Therefore, to achieve useful fast
The Author and good models, we need to take a more thoughtful approach.
Imagine that every governing equation—whether it be
Marlantes related to ? uid ? ow, structural deformation, or dynamics—
Kyle E. Marlantes is a naval architect, software could be decomposed into two parts: a low-cost, low-? delity developer, and PhD candidate at the Univer- part, and then a high-cost, high-? delity part. In fact, this is a sity of Michigan, where he develops methods to leverage data in engineering applications.
very natural idea in engineering: take the complex, close-to- life model, and make assumptions to yield a model that is trac- table. Traditional methods, which still comprise much of the bulk of engineering analysis today, would simply throw out the high-? delity part and just solve the low-? delity part, and do so at a great cost savings. For many problems, the low-? - delity part also tends to be robust: it often captures most of the solution. But what if we modeled the high-? delity part using machine learning? The result is a model which is composed of both physics-based and data-driven parts—a hybrid method.
While talk of generative AI and large language models rag- es on, when it comes to engineering analysis, the real gem is hybrid methods. Though the idea is very new—even to the research world—hybrid methods are demonstrating a few key characteristics: ? rst, methods of this type greatly reduce the amount of training data required. In general, the amount of data required is a small fraction of what data-only methods need. Second, hybrid methods are almost as fast to evaluate as purely data-driven methods, because the low-? delity physics part of the model usually requires minimal computational re- sources. Third, hybrid methods still yield solutions with accu- racy similar to that of the training data. This means that if we use a small amount of costly high-? delity simulation data or model test data, we can make similar-? delity predictions with the resulting hybrid method. And lastly, but most importantly, hybrid methods are being shown to be transferable. Continu- ing with our seakeeping example, this means we can take that one seaway worth of high-? delity data and make similar-? - www.marinelink.com 15
MR #9 (1-17).indd 15 9/6/2023 4:36:52 PM