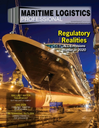
Page 18: of Maritime Logistics Professional Magazine (Nov/Dec 2019)
Short Sea Shipping Ports
Read this page in Pdf, Flash or Html5 edition of Nov/Dec 2019 Maritime Logistics Professional Magazine
ENVIRONMENTAL REVIEW
Avoiding a Wrong Turn to
IMO Compliance
By Simon Whitford
Machine learning is the clever part of this story but accessing vessel data is also important.
Equipping vessels with ioT sensors/dataloggers has become a cheap, straightforward business which does not require vessel downtime.
Only small packets of data are required and transmissions costs are modest and falling.
Using data to optimize speed n October, the International Maritime Organization faced calls for a blanket 20% reduction of vessel speed in order to reduce Speed optimization cuts GHG emissions without affecting
Igreenhouse gas emissions. While the proposal was ultimately transit times. Along a given route, departure and arrival dates, a not carried forward at that conference, slow steaming is still com- machine learning enabled ship saves fuel by adjusting speed to mon among shipowners in response to poor demand and the low take best advantage of a host of forecast environmental factors. rates of utilization endured since 2008. To enable vessels in this way, Owners and Charterers use ma-
Whilst cutting the speed of ships does help reduce emissions, chine learning to create and then continuously refne each vessel’s vessel speed is only part of the solution and a simple speed limit unique performance model. is not practical across the board. To reduce emissions and cut fuel For such vessels, the operator can then accurately deconstruct waste reliably, ship owners and charterers must optimize vessel each element of the vessel’s GHG emissions – separating out speed across each voyage – optimization being quite different weather, trim, fouling and speed effect to reveal a dynamic base- from a blanket speed limit. line – the vessel’s underlying level of performance.
Machine learning is the clever part of this story but accessing vessel data is also important. Equipping vessels with ioT sensors/
Slow steaming is not the answer
Slow steaming may reduce the emissions of a given vessel and dataloggers has become a cheap, straightforward business which each vessel has its “most economical” sweet spot speed, but a does not require vessel downtime. Only small packets of data are slower voyage needs more vessels to meet the same level of de- required and transmissions costs are modest and falling. mand. More ships mean more cargo in transit and a one off “stock- Through this route, machine learning enabled vessels with dy- ing” boost in global factories to fll this “fatter” supply chain. As a namic consumption baselines have access to a range of optimiz- result, both factories and shipyards would experience a short-term ing and decision support tools, notifcations, analytical methods increase to production to fll these vessels and this spike in manu- and push reports which together drive improvements in vessel facturing activity would inevitably add to carbon emissions. performance across all stakeholders on board and onshore.
In practice, more vessels could worsen port congestion, and a To plan a voyage for a machine learning enabled vessel, hun- mandatory speed cap might prevent vessels taking measures to dreds of thousands of speed profle simulations are run along a avoid bad weather en-route. Slow steaming has already created particular route – each one predicting the vessel’s response to the technical issues like cold corrosion of marine engines. latest forecasts of weather, sea state, and so on. The speed pro- 18 Maritime Logistics Professional November/December 2019 | |